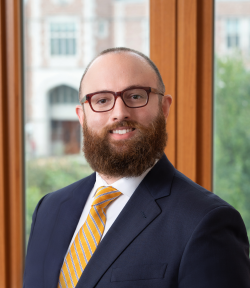
Landon J. Ross
About
I am an assistant professor at Tulane University. I work in the finance department for the A. B. Freeman School of Business. My research interests include applications of machine learning, large-scale optimization, robust optimization, and natural language processing in finance. My main research field is asset pricing.
I was previously a financial economist at the U.S. Securities and Exchange Commission in the Office of Markets. I completed my Ph.D. in Finance at the Washington University in St. Louis Olin Business School. Before starting my Ph.D., I worked as a research assistant at the Federal Reserve Board of Governors.
Papers
- Risk Exposures from Risk Disclosures: What They Said and How They Said It
- with Seth Pruitt and Rahul Mazumder
- Revise and Resubmit at Journal of Financial Economics.
- SSRN
- Bottom Up vs Top Down: What Does Firm 10-K Tell Us?
- With Jim Horn, Kaihong Luo, Mert Pilanci, and Guofu Zhou
- Revise and Resubmit at Journal of Financial Markets.
- SSRN
- Cash-Hedged Stock Returns
- with Chase Ross and Sharon Ross
- Revise and Resubmit at Review of Asset Pricing Studies.
- SSRN
- Expected Returns, Firm Characteristics, and Cardinality Constraints
- SSRN
- Are characteristic interactions important to the cross-section of expected returns?
- SSRN
- Are Item 1A Risk Factors Priced?
- SSRN
- Generating options-implied probability densities to understand oil market events
- Energy Economics (2017)
- with Deepa Dhume Datta and Juan M. Londono
- SSRN
- A Facilitated Interface to Generate a Combined Textual and Graphical Database System Using Widely Available Software
- Journal of Software Engineering and Applications (2012)
- with Corey Lawson, Kirk Larson, Jonathan Van Erdewyk, Christopher Smith, Al Rizzo, Marc Rendell
- Link